The AI factor
Quantitative Investments
As we have seen in our previous Expl(AI)ning piece, factors have been the backbone of quantitative equity investing—offering a structured way to explain returns that once seemed random. But as their popularity grew, so did their limitations. The lack of a dynamic timing model, the imposed linear constraints, and rigid assumptions, have diminished the glamour of factors.
What if we could break free from these constraints? Instead of forcing markets to fit predefined factor models, is there a way to make generic models understand markets and capture their complexity? AI provides a solution here, in that it provides us with new techniques to extract signals from data. Also, by embracing adaptability, non-linearity and predictive power, AI-driven systems have the potential to redefine how we use factors—turning them from static descriptors into forward-looking indicators.
In this Quanta Byte of the Expl(AI)ning series, we provide an example about how this could be achieved. We introduce the notion of AI-powered factors and present a framework for how AI could revolutionize factor investing.
AI powered factors
At Vontobel, we continuously refine our approaches by merging traditional financial analysis with cutting-edge technological innovations. In our previous discussions, we underscored the importance of the quality factor as a fundamental descriptor and driver of stock performance. Today we build on that narrative by introducing an AI-powered quality factor.
The basic idea is that we trained our AI system to forecast a company’s future quality, and we built portfolios based on that. The logic behind the idea is that if quality works, we’d like to be holding positions in companies whose quality is not just good but stays good and even improves.
This article is organized in three parts. First, we provide a framework on how to time factors, which we saw is crucial to success at factor investing. Second, by leveraging the nonlinear interactions hidden within the data, we show our AI system captures relationships that are non-linear. Third, we generate forecasts that outperform the naïve forecasting approach embedded in factor investing, as discussed in the previous piece. In doing so, we overcome the limitation of linear factor frameworks.
AI takes care of timing
Our AI system takes care of time in two ways. First, as time goes by, it continuously adds data to the training set. Second, it re-learns frequently.
Figure 1 illustrates how this works in practice. Imagine that ‘now’ is denoted as t, as indicated in the figure. Since our AI system forecasts quality one year ahead, we start by removing the last year of data. Quality yesterday needs to be given to an AI system that only knows stock characteristics as of yesterday minus one year. Not doing so would be ‘cheating’. The year of data that’s removed is denoted by the dark green shaded area in the figure. We then let the model run ‘live’ for a period. After that, we are at t+1 and repeat the process. Note that ‘Train Data 2’ is larger than ‘Train Data 1’ because we add data for the period that just passed (from t to t+1).
This way of dealing with data is called expanding window approach. The idea is that we want to be able to learn from the largest amount of data possible at any point in time.
An alternative way of doing things would be to ‘roll’ a fixed-length time window as time goes by. To illustrate how this works, assume ‘Train Data’ 1 is 10 years long. When the next period comes (t+1), we would still look at only the last 10 years of data. If you assumed that our period is one month long, this is equivalent to saying that we would build ‘Train Data 2’ by discarding the first month of data in ‘Train Data 1’ and adding the last month (from t to t+1).
Rolling windows were standard practice in a non-AI world because quantitative methods were not capable of handling excessively large datasets. Let us elaborate. If all you have is a linear regression, and your decisions are based on the slope of the same, you want the slope to be reflective of the times you’re living. If you fed your linear regression model with 10 years of data, and continue to expand, you’ll get a result that may work for 10 years (and more) but may not help you navigate markets next month. To solve these problems, practitioners often chose the look-back period of rolling windows to strike a compromised between genericity (advocating for long windows) and specificity (advocating for short windows). Since AI can solve for this, why bother making this arbitrary choice? Let AI decide, and let’s give it all the data we have.
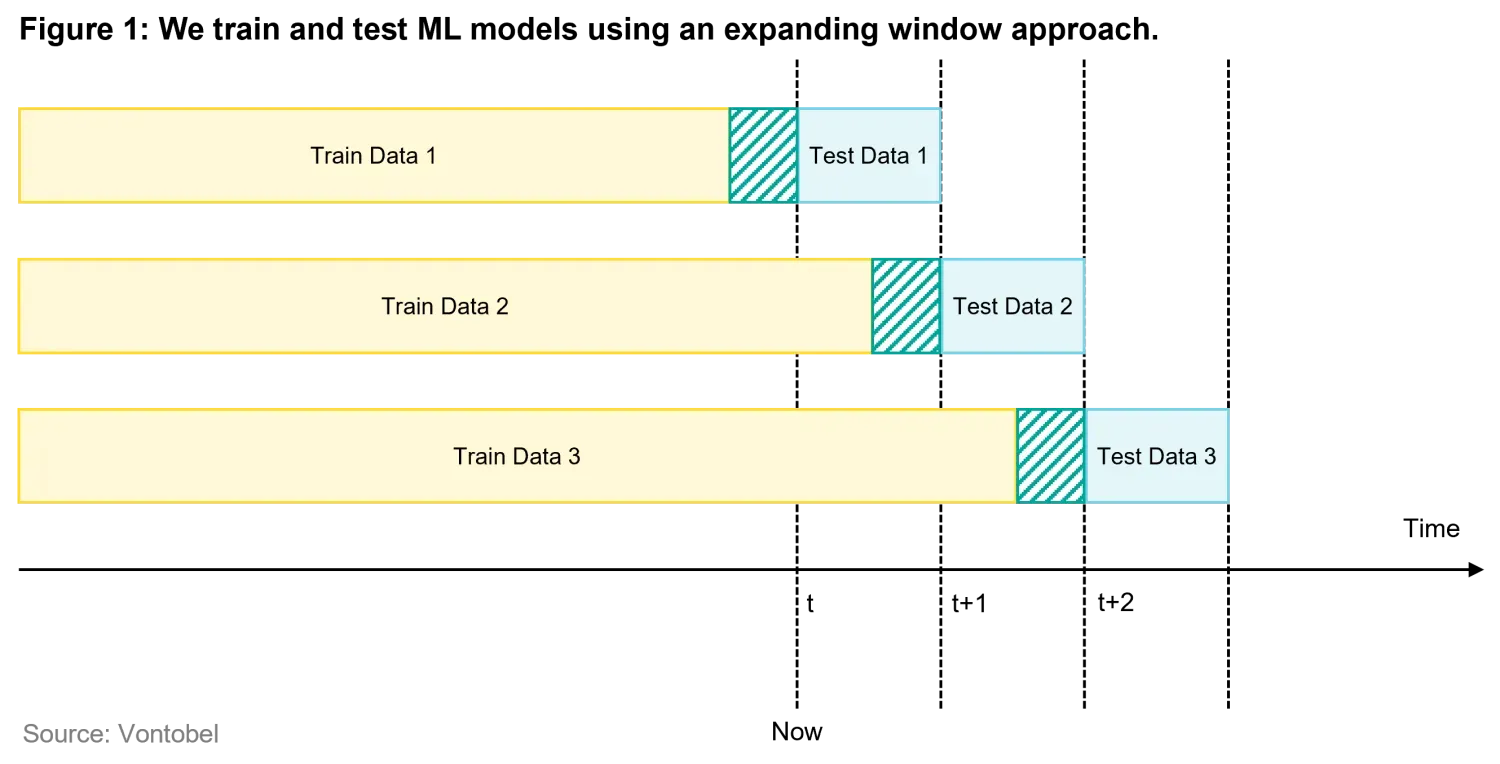
AI can exploit non-linearities
Our AI system goes beyond traditional linear assumptions. In fact, our AI system does not postulate *any* model structure, linear or non-linear. It lets the data speak about what to do. Thus, it allows us to capture complex, non-linear interactions among market variables. Consider Figure 2 for example, where EPS growth (in % terms on the x-axis) is plotted against its relevance (on the y axis). As one can deduce by simply inspecting the figure, the relationship between the two is clearly non-linear, which makes sense intuitively. If EPS growth is very low, it does not matter if it’s even lower. There is simply no EPS growth. If EPS growth is very high, it does not matter much if it’s higher. AI is telling us that there is a zone in between where changes in EPS growth have a big impact on the stock’s future return potential.
Figure 2 also shows us the perils of imposing a linear relationship to the data. Assume we fit a linear regression to the curve, akin to what factor models do, and represent that on the chart (coral line). One sees two drawbacks. First, we assume a linear relationship where none is present (at the low and high end of the chart). Second, the relationship in the area ‘in between’ is less reactive than it should be (the coral line is less steep than what suggested by the cloud of green dots in the ‘in between’ area).
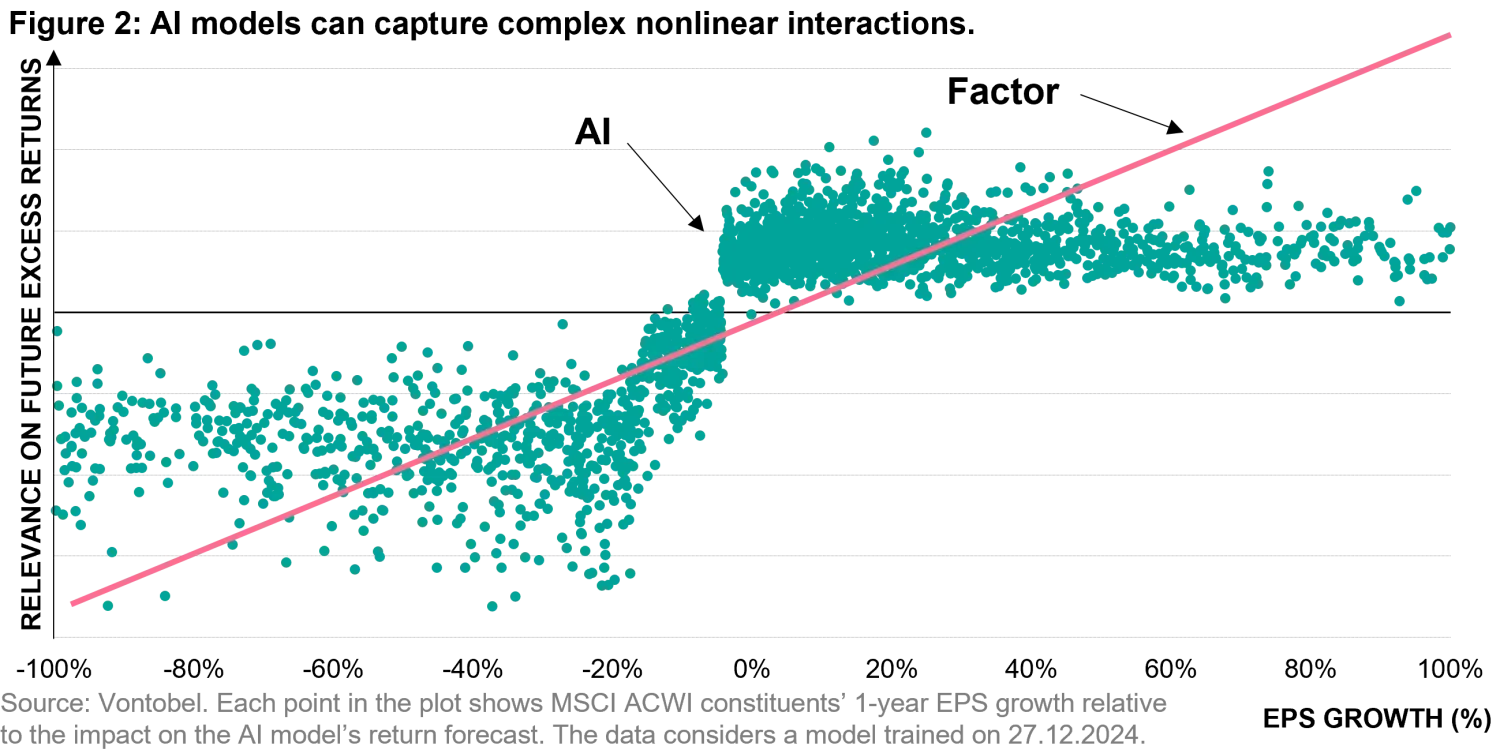
AI produces actual forecasts
Our AI system is built for forecasting. This seems obvious, but classical factor investing does not rely on forecasts. If investors say that they invest along quality, they mean that they invest in companies that have high quality today. They do so in the hope that high quality today means good returns tomorrow. As we will see shortly, this is not a bad assumption ‘per se’, but it’s not traditional forecasting. It’s ‘naïve’ forecasting.
Imagine it’s raining today, and you want to forecast the weather tomorrow. ‘Naïve’ forecasting would mean that you forecast rain for tomorrow, since it rained today. This is not a bad way to forecast, as weather patterns tend to correlate. However, you can do better and consult professional weather forecasting systems.
There is a special sauce in the way we do forecasting here. Instead of treating factors as static descriptors of a company's current state, our approach transforms them into dynamic forecasts—anticipating how key characteristics will evolve and influence future returns. This predictive capability enhances the factor’s effectiveness, making it not just an explanatory tool but a forward-looking signal for better investment decisions.
As shown in Figure 3 below, this approach leads to consistently lower forecasting errors compared to a naïve model, demonstrating how AI-driven predictions provide a more accurate and reliable view of future market conditions than simply looking at the current state of the market. The improvement is not only significant, but persistent over time, as indicated by the fact that the relative difference between the curves remains constant over time.
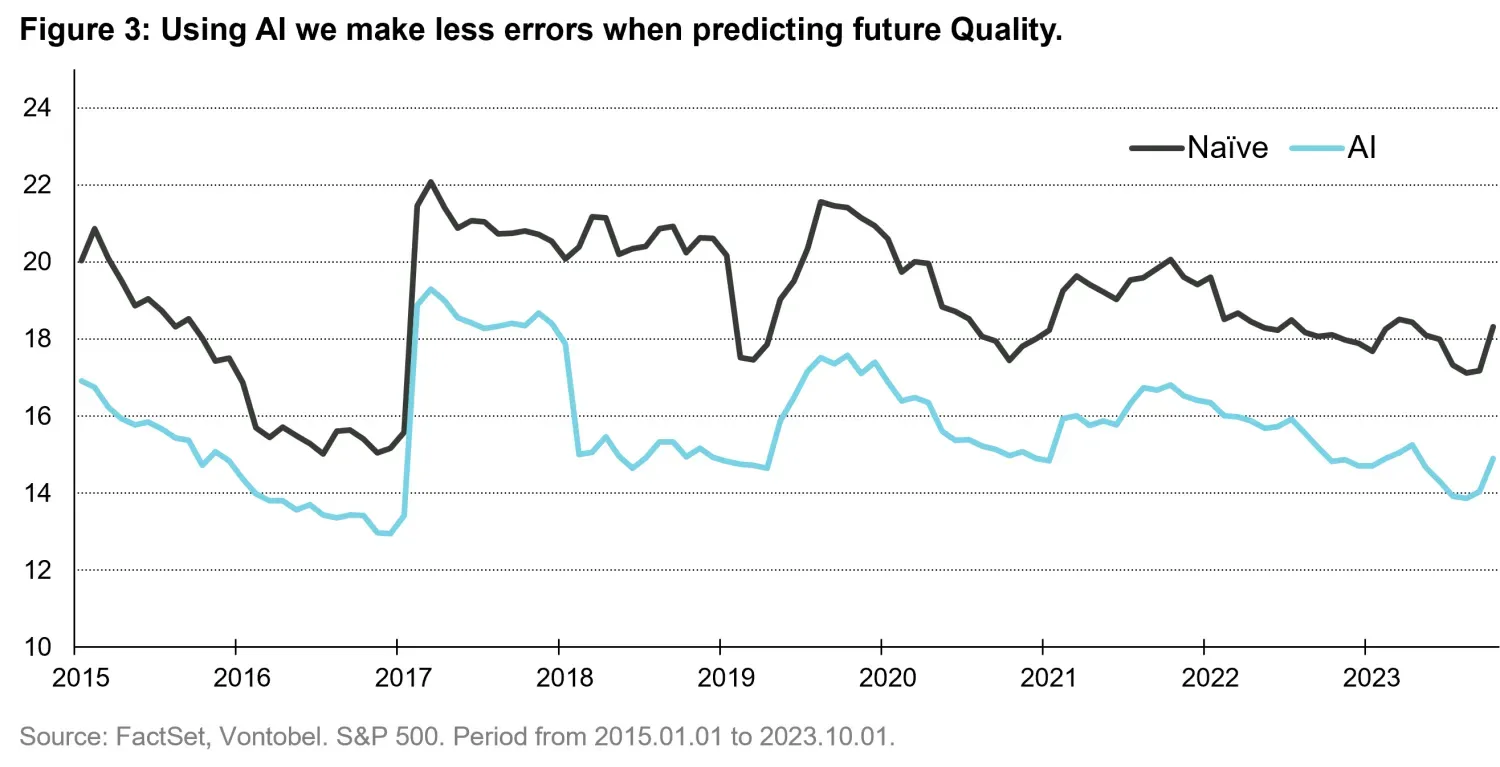
Overall impact
To assess the effectiveness of our AI system when forecasting the quality factor, we analyze its impact through a transition matrix, similarly to what we did in the previous article. For a detailed walk-through of how to read Figure 4 please refer to the discussion contained therein. This visualization tracks how companies move across quality categories over time. This provides a clear picture of how well the model identifies persistent quality characteristics and anticipates shifts in company fundamentals. Compared to the naïve approach—where investment decisions rely solely on the current quality positions—the AI-powered quality factor demonstrates a more forward-looking perspective, capturing subtle signals that indicate future changes.
By examining the transition matrix in Figure 4 below we measure that in this case AI is more accurate at forecasting the transitions that matter. We indicate with green the transitions that AI has predicted more accurately. In yellow the ones that are unchanged and in red those that got worse.
Only 6 out of the 25 cells in Figure 4 have a red arrow. Said differently, AI improved outcomes in 76% of the cases (19 cells out of 25). Also, note that the ‘best’ columns (defined as those where AI led to worse or equal forecasting in only one cell in the column) are the first and the last. These correspond to companies that ascended to ‘Very good’ or descended to ‘Very bad’ in the period respectively. On average, the AI system can reduce the error by cutting it in half. These kinds of improvements are what makes a difference when the signals are implemented in a large, diversified equity portfolio.
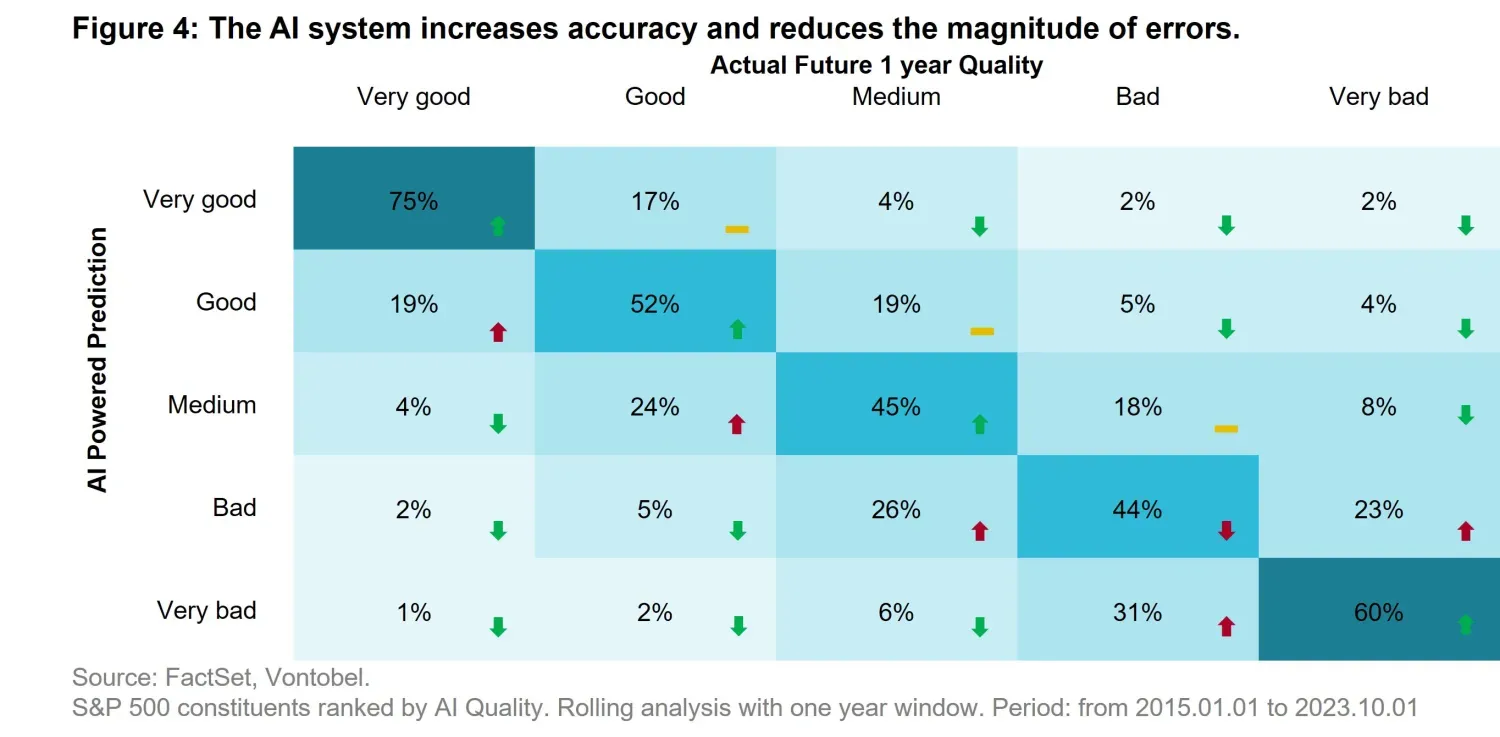
Conclusion
AI-driven factor investing is not just an improvement—it’s a fundamental shift in how we approach systematic equity strategies, and we are sure you’ve heard that statement before. However, this paper shows you a more nuanced, yet equally powerful truth. Very often AI practitioners advocate for a blind utilization of AI, one which would create a tabula rasa of previously adopted approaches (like traditional factor investing). Along those lines, one would simply forget about factors and use AI from scratch. While legitimate, this approach misses the fact that AI is in fact so flexible, that it can improve factor investing without challenging its underlying principles (which we find useful).
Our framework has shown that AI can address the key pitfalls of traditional factor models, turning their limitations into strengths. By dynamically adjusting to market conditions, identifying non-linear relationships, and improving predictive accuracy, AI enhances both the robustness and the forward-looking nature of factor investing.
What makes this approach even more powerful is its flexibility—while we demonstrated it with the quality factor, the same methodology can be applied across other factors, opening the door to a broader transformation. Just as the world once debated whether all cars would eventually be electric—and today we see that shift becoming reality—the same evolution is happening in factor investing. The future is AI-powered.
But what about explainability? A common concern is that AI systems function as black boxes, making them difficult to interpret. Why is the AI system ranking this stock so well? These are the type of questions that we would like to be able to answer as investors. In our next discussion, we’ll explore how modern AI techniques allow us to bring transparency to these systems, demystifying their decisions and ensuring that investors can trust the insights they provide.
Important information: This content is for informational and educational purposes only to provide a framework to assist in the implementation of an investor’s own analysis and an investor’s own view on the topic discussed herein. Nothing is or should be construed as an offer to sell, or a solicitation of an offer to buy, any security or other instrument. Vontobel makes no representations, express or implied, regarding the accuracy or completeness of this information which can change at any time without notice. Opinions expressed herein may be those of individuals and may differ from the views and opinions expressed by Vontobel; current as of the date indicated and are subject to change. This material may contain forward-looking statements, which are subject to uncertainty and contingencies outside of Vontobel's control. Recipients should not place undue reliance upon these forward-looking statements. There is no guarantee that Vontobel will meet its stated goals. Past performance is not indicative of future results. Investment involves risk, losses may be made. The term "AI System" and 'Our AI" as used herein stands generically for a theoretical view of AI by Vontobel, and is used to illustrate how Vontobel approaches the topics discussed. The methods and models as described do not refer to any strategy offered, and the content should not be understood as a description of any models and methods currently used by Vontobel" MSCI Inc’s data and analytics were used in preparation of this information for illustrative purposes only.. Copyright 2024 MSCI, Inc. All rights reserved. While the information herein was obtained from or based upon sources believed by the author(s) to be reliable, Vontobel has not independently verified the information and provides no assurance as to its accuracy, reliability, suitability or completeness.